Improving conversational artificial intelligences or simpler prediction engines involves overcoming biases, that is, going beyond the limits of data. But the notion of bias is subtle, as it depends on the goals.
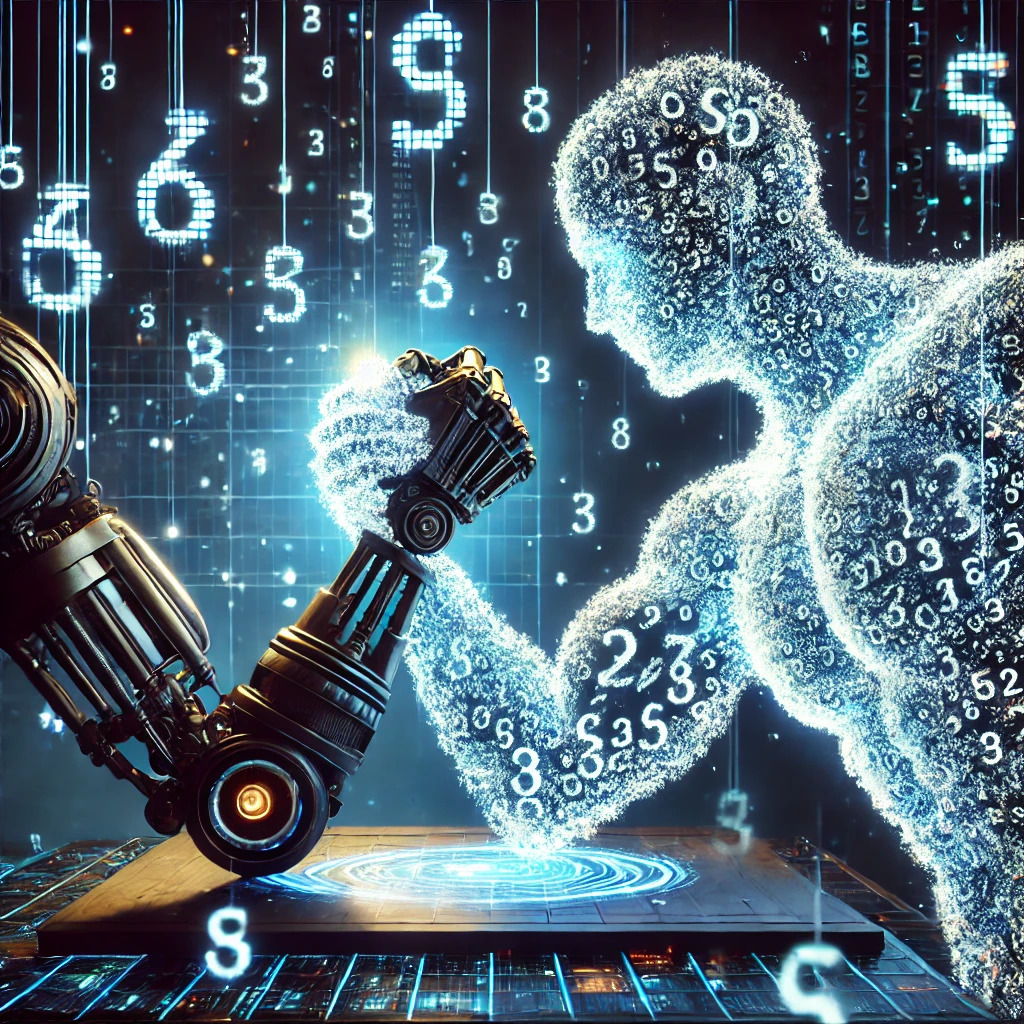
Note
This post was originally published in French as part of my scientific chronicle in Les Echos.
In 2023, Microsoft’s conversational AI insulted users. Salary-recommendation engines ignore women’s degrees to underpay them. At the start of the Covid-19 pandemic, predictions of hospital stays consistently underestimated the duration. These three issues all stem from the same failure: predictive engines, artificial intelligences, that have learned from biases. The rude conversational AI replicated its training texts, some of which came from internet forums where politeness is sometimes overlooked. The medical AI only considered finished hospitalizations, and, as the epidemic had just begun, only patients with mild forms had already been discharged, while the more seriously ill remained hospitalized.
To obtain an AI that doesn’t say nonsense, the biases must be “corrected.” The problem of too-short observation windows is a classic issue in medical statistics: more importance must be placed on the few individuals who have been sick for a long time. A similar solution is used to improve conversational AIs: weighting the training text sources based on the deviation from the desired behavior.
Aligning on which values?
The problem of bias is universal in statistics. And modern AIs are statistical because they learn from data. The notion of bias is very relative. It should be understood as a gap between the available data and the desired behavior. Therefore, there is no such thing as unbiased data, or a universal bias correction. Much of the effort to improve AIs focuses on reducing this gap between training and the desired behavior.
For example, when training AIs for autonomous vehicles, one difficulty is that the data contains very few traffic accidents. Simulators are sometimes used to fill this gap. They are inherently less rich than reality and are mixed with real-world driving. There is a well-controled gap between the resulting mixture and typical driving, this gap is there to put emphasis on safety requirements in unfavorable scenarios. This is another form of data correction.
Just as the notion of data bias depends on how well the data match a targeted use, an AI does not produce absolute or objective truth. Without corrections, it simply replicates its behavior based on what it has observed. And when corrections are made, the whole question is how to correct it. For powerful AIs, we then talk about “alignment” towards goals and values. As AI incorporates the values of its designers, one might wonder whether the same AI can be socially acceptable in all cultures.
AI chronicles
Find all my AI chronicles here
The goals of these “AI chronicles” is to introduce concepts of AI to a broader public, staying at a very very high level.