Note
Click here to download the full example code or run this example in your browser via Binder
2.2. Interpreting random forests¶
Interpreting random forests
2.2.1. Data on wages¶
We use the same data as in the linear-model notebook
import os
import pandas
# Python 2 vs Python 3:
try:
from urllib.request import urlretrieve
except ImportError:
from urllib import urlretrieve
if not os.path.exists('wages.txt'):
# Download the file if it is not present
urlretrieve('http://lib.stat.cmu.edu/datasets/CPS_85_Wages',
'wages.txt')
# Give names to the columns
names = [
'EDUCATION: Number of years of education',
'SOUTH: 1=Person lives in South, 0=Person lives elsewhere',
'SEX: 1=Female, 0=Male',
'EXPERIENCE: Number of years of work experience',
'UNION: 1=Union member, 0=Not union member',
'WAGE: Wage (dollars per hour)',
'AGE: years',
'RACE: 1=Other, 2=Hispanic, 3=White',
'OCCUPATION: 1=Management, 2=Sales, 3=Clerical, 4=Service, 5=Professional, 6=Other',
'SECTOR: 0=Other, 1=Manufacturing, 2=Construction',
'MARR: 0=Unmarried, 1=Married',
]
data = pandas.read_csv('wages.txt', skiprows=27, skipfooter=6, sep=None,
header=None)
short_names = [n.split(':')[0] for n in names]
data.columns = short_names
# Log-transform the wages, as they typically increase with
# multiplicative factors
import numpy as np
data['WAGE'] = np.log10(data['WAGE'])
features = [c for c in data.columns if c != 'WAGE']
X = data[features]
y = data['WAGE']
2.2.2. Feature importance¶
from sklearn import ensemble
forest = ensemble.RandomForestRegressor()
forest.fit(X, y)
# Visualize the feature importance
importance = forest.feature_importances_
from matplotlib import pyplot as plt
plt.barh(np.arange(importance.size), importance)
plt.yticks(np.arange(importance.size), features)
plt.tight_layout()
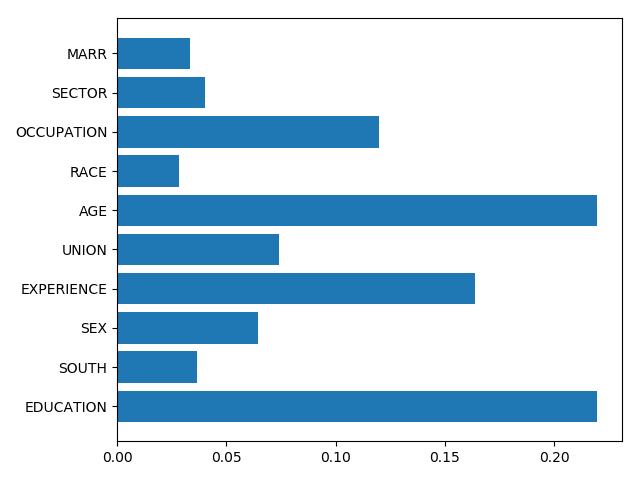
2.2.3. Meaning and Caveats¶
Feature importances are a proxy for the mutual information between the feature and the target, conditionally on the other features. The conditioning is difficult and not well controlled.
Higher-cardinality categorical variables will have larger feature importances
Total running time of the script: ( 0 minutes 0.086 seconds)